High Precision map automation Lane Vectorization
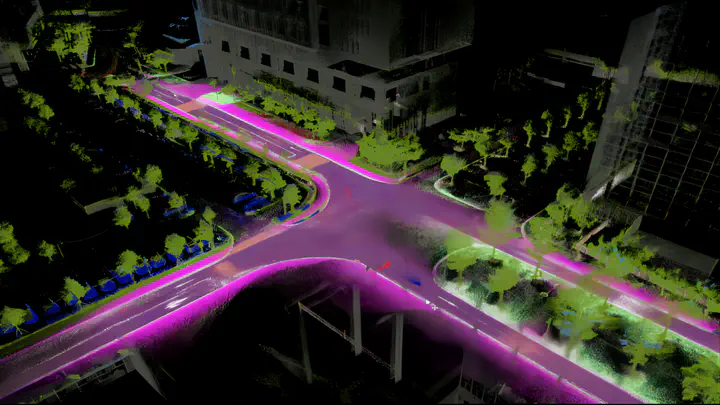
Based on the Mask2Former network, semantic segmentation was performed on the image data, and 3D lane line point clouds were extracted through the camera and LiDAR extrinsics. Finally, the 3D lane lines were clustered and curve-fitted, achieving high-precision map automatic vectorization of lane lines.
Our manual handle data record platform:
In order to quickly verify the algorithm , we built our owndata record platfor(handle & package). After many iterations, now out platform can compatibility a variety of lidar(Tested:Hesai32、Robosense32、Livox mid70…), IMU(Tested: Xsens mti710、Lpms ig1) and camera(Hikvision、Intel D435i). We also carried out hardware synchronization and external parameter calibration on our equipment.
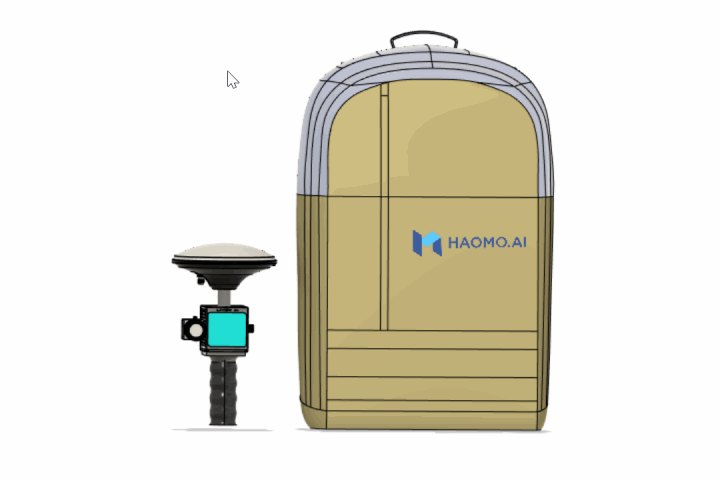
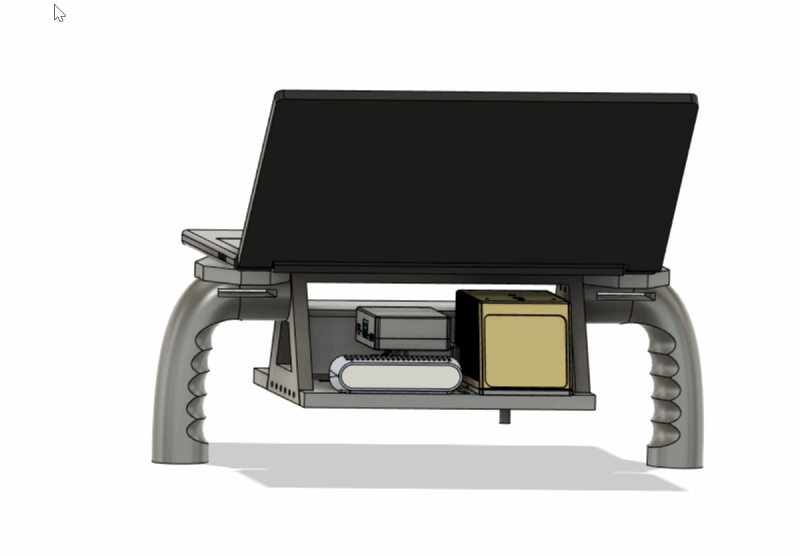
manual data record platform
pps hardware synchronization
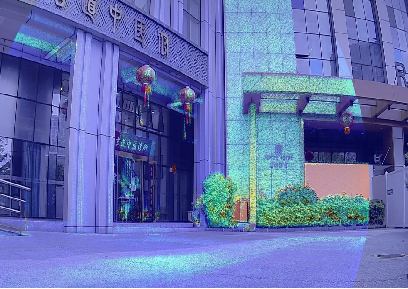
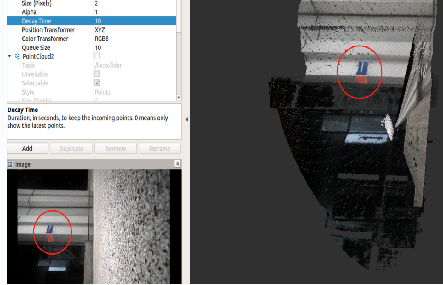
Automatic calibration of external parameters
Mapping:
Based on FASTLIO-SAM .Construct pointcloud map, area:1km
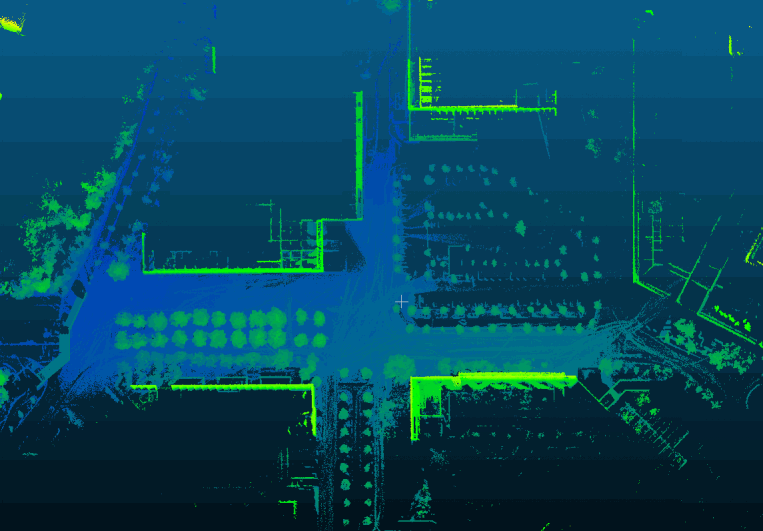
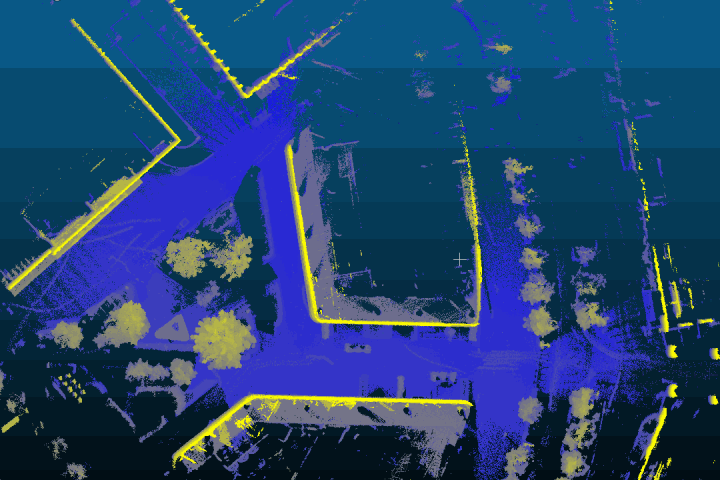
Color Mapping:
RGB Pointcloud map is constructed through the external parameters of the camera and lidar
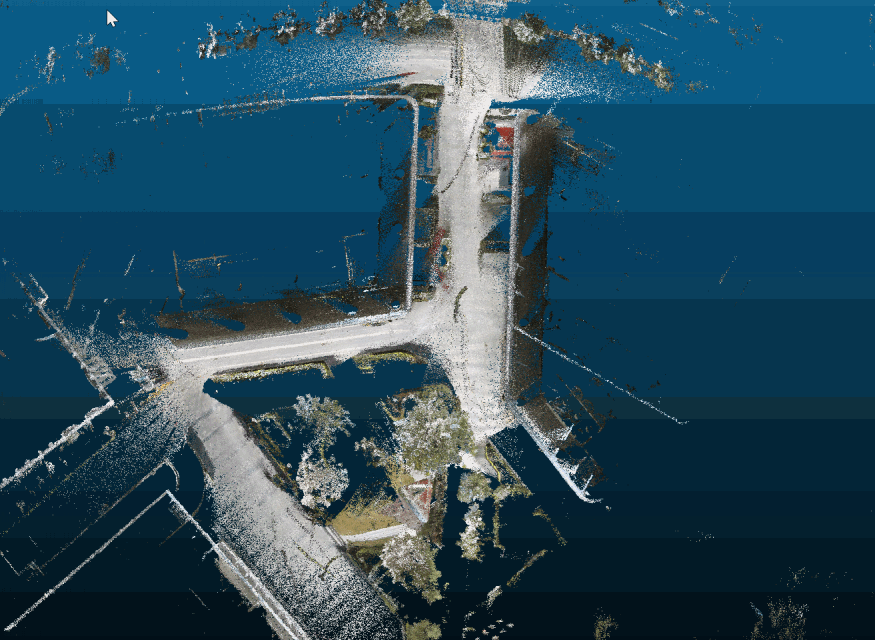
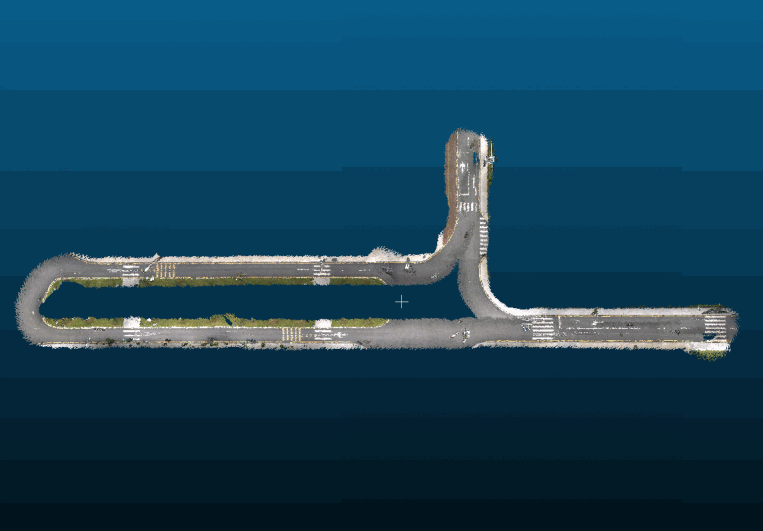
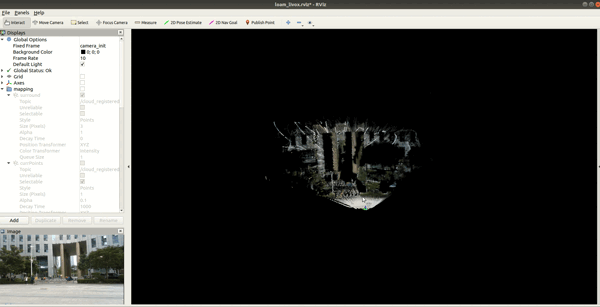
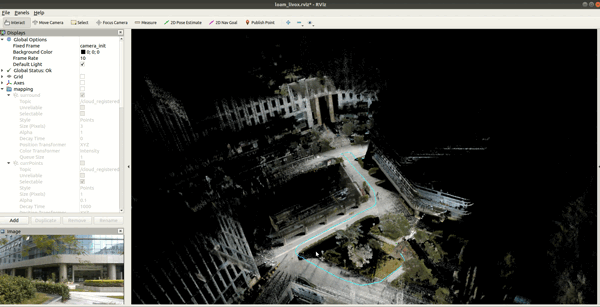
Semantic Map:
Semantic Pointcloud map is constructed through the external parameters of the camera and lidar
Road semantic segmentation based on Mask2Former model
3D Semantic Map
Extraction of ground elements:
Lane vectorization: